Smart Advertising: AI-Driven Innovation and Technological Disruption in the Advertising Ecosystem
Abstract
Introduction: The impact of artificial intelligence (AI) in media industries has received increasing attention in recent years. Research literature in this regard has focused on content production and distribution sectors, leaving advertising in a discreet background. This article aims to take the study of AI and advertising to the forefront, identifying the scope of current research on the subject and offering a map of the research trends, drawing them as a key vector of technology-based innovation in the media ecosystem. Methodology: In order to do so, a qualitative scoping review of the current research literature on AI and advertising has been developed, completed by the contribution of professional reports from the sector. Results and discussion: The existing literature points to the efficiency in large unstructured data sets processing, predictive / prescriptive analysis, natural language recognition and image recognition, as automation as the main AI innovation vectors. The disruptive impact of AI affects all phases of the advertising process: market research and analysis, creativity, media planning and buying, and effectiveness evaluation. Conclusions: Research tends to perpetuate the traditional structure of the advertising process and obviates the ecosystem dimension of innovation, which transforms actors and their relationships.
KEYWORDS: artificial intelligence; advertising; programmatic; creativity; automation; deepfake; big data.
Smart Advertising: Innovación y disrupción tecnológica asociadas a la IA en el ecosistema publicitario
RESUMEN
Introducción: El impacto de la inteligencia artificial (IA) en las industrias del contenido y la comunicación es objeto de atención creciente en los últimos años. La literatura y las investigaciones en este sentido han tendido a centrar el foco en los sectores vinculados a la producción o distribución de distintas formas de contenido. La publicidad, sin embargo, ha permanecido en un discreto segundo plano. Este artículo pretende sacar de ese segundo plano al estudio de la IA en publicidad, identificar la orientación y alcance de las investigaciones recientes sobre el tema y ofrecer un mapa de las líneas de investigación en desarrollo, acotándola como un vector clave de innovación de base tecnológica en el nuevo ecosistema mediático. Metodología: Para ello se ha desarrollado una revisión sistemática exploratoria cualitativa (qualitative scoping review) de la literatura de investigación sobre IA y publicidad, completada por la aportación de informes profesionales del sector. Resultados y discusión: La literatura existente apunta a la eficacia en el procesamiento de grandes conjuntos de datos desestructurados, el análisis predictivo / prescriptivo, el reconocimiento del lenguaje natural y de las imágenes y la capacidad de automatización de tareas como principales vectores de innovación de la IA. El impacto disruptivo de la IA afecta a todas las fases del proceso publicitario: investigación y análisis de mercados, creatividad, planificación y compra de medios y evaluación de la eficacia. Conclusiones: Las investigaciones tienden a perpetuar la estructura tradicional del proceso publicitario y obvian la dimensión de ecosistema de la innovación, que transforma a los actores y sus relaciones.
PALABRAS CLAVE: inteligencia artificial; publicidad; programática; creatividad; automatización; deepfake, big data.
This work is part of the R+D+I project Innovation ecosystems in the communication industries: Actors, technologies, and configurations for the generation of innovation in content and communication (INNOVACOM), with reference PID2020-114007RB-I00 financed by the Ministry of Science and Innovation AEI/10.13039/501100011033
How to cite this article / Standard reference
Martínez Martínez, I. J., Aguado Terrón, J. M. y Sánchez Cobarro, P.H.. (2022). Smart Advertising: AI-Driven Innovation and Technological Disruption in the Advertising Ecosystem. Revista Latina de Comunicación Social, 80, 69-90. https://www.doi.org/10.4185/RLCS-2022-1693
Translation by Paula González (Universidad Católica Andrés Bello, Venezuela)
Keywords
artificial intelligence, advertising, programmatic, creativity, automation, deepfake, big data
Keywords
inteligencia artificial, publicidad, programática, creatividad, automatización, deepfake, big data
Introduction
The impact of artificial intelligence (AI) in the content and communication industries has received increasing attention in recent years. The processes subject to innovation due to the influence of AI include the automation of writing or production tasks (Túñez-López et al. 2018), the optimization of Big Data in profile analysis or inventory management (Napoli, 2014), the transformation of formats and channels, or the management of communications with the user (Huang and Rust, 2021). Literature and research in this regard have tended to focus on the sectors linked to the production or distribution of different forms of content. Particularly intense is the interest in the impact of AI in journalism, a continuation of an analytical tradition -that of data journalism- that connects with a current problem of a strongly transformative nature: disinformation (Ali and Hassoun, 2019; Manfredi and Ufarte, 2020). Advertising, however, has remained a discreet background in the mainstream literature on AI and the media. And this is despite the importance given in the field of communication to technology-based innovation (Allan, 2016). If we consider advertising as the most relevant business model of the second half of the 20th century and artificial intelligence as the most disruptive technological development of the first half of the 21st century, it is surprising how little treatment has been received by a transformation that undoubtedly has a backbone of the media industry.
Consequently, we consider the impact of artificial intelligence in advertising to be a key element of the digital transformation of the media ecosystem for at least three reasons:
In the first place, it concerns the transformation of the backbone business model of the ecosystem of both traditional media and the Internet of platforms: the monetization of attention (Arvidsson & Colleoni, 2012). Advertising still constitutes the only viable alternative, together with the exploitation of personal data, to the model of payment for access to content. In fact, besides the monetization of attention, advertising is also one of the main ways of monetizing personal data (Acquisti et al., 2016).
Second, the technological change, in the case of advertising, precedes and exceeds in intensity that of conventional content industries, with the sole exception of native digital industries, such as video games (Lee and Cho, 2020). The experts consulted in previous fieldwork (Martínez and Aguado, 2019) point out, precisely, how the pressure of digitization reaches the agencies before it reaches the media and how those furthest behind incorporate experiences from native digital industries. Among the reasons for this differentiation, two are fundamentally pointed out: the greater proximity/sensitivity to users, whose daily life and preferences are the object of constant scrutiny in advertising research; and the early incorporation of Big Data into the research and analysis phases of advertising, which favors a growing demand for digitization (Ibid.).
And, finally, the third reason why the study of the impact of AI in advertising is of strategic interest is that impact is at the epicenter of one of the central issues of the digital society of the 21st century: the problem of privacy (Acquisti, 2016; Li, 2019). In fact, social awareness of privacy protection in the digital environment has grown associated with the consolidation of data exploitation as the backbone of the transformation of advertising (Malthouse and Li, 2017). The pressure of this growing sensitivity has led to the development of policies and regulations on privacy and the use of digital data, as well as the positioning of the main actors and the development of a strategy on the problem of privacy. The latest disruptive movement in this regard has been the so-called “cookie apocalypse”, which indicates the abandonment around 2023 of third-party cookies as the main tertiary data collection system (Barker, 2020). This movement represents a radical transformation of digital advertising (hence the mention of the “apocalypse”) in which AI has a role as a factor in possible solutions to the dilemma of how to collect and process relevant information about user behavior without affecting privacy (Buckley, 2021).
Objectives
This article aims to remove the study of AI in advertising from the background, responding to its condition as a structuring business model of the digital content ecosystem, whose transformation determines the viability of content industries in the process of adaptation.
For this, it is necessary to carry out, in the first place, an analysis of the existing research on AI and advertising, considering its dimension, scope, and characterization. Additionally, from the review of the existing literature, it is intended to identify the dominant topics, approaches, and conceptual frameworks.
From the catalog of topics and objects of interest in the field of the impact of AI in advertising, it is also possible to delimit the horizon of challenges and future lines of research that current research works envision. Along with this, it will also be possible to draw a table of gaps and absences that allows proposing complementary lines of research, thus proposing a specific research program that connects the innovative contribution of AI in advertising with the process of change in the advertising ecosystem and its connection with the challenges and problems of digital content industries.
The research questions that structure the selection and analysis of the sample are:
1) What definitions and/or context and/or projection of the relationship between AI and advertising does the research provide?
2) What features or capabilities of AI drive innovation in the advertising ecosystem?
3) What aspects of advertising does the impact of AI transform and how does it transform them?
Methodology
To answer the research questions, a qualitative scoping review has been developed. Unlike traditional systematic literature reviews, according to Grant and Booth (2009, p. 95), qualitative scoping reviews constitute a method of integrating or comparing the results of qualitative studies, identifying those topics or approaches that are transversal to different works. The exploratory nature seems particularly suitable in the case of a preliminary approach to an emerging field of study (Codina, 2020).
In this research, the four phases established by the so-called SALSA framework (search, appraisal, synthesis, analysis) (Codina, 2020) have been applied to a sample of quality scientific production (articles, book chapters, and books with a blind peer-review guarantee) circumscribed to 2016-2021 for reasons of thematic opportunity (greater probability of the presence of relevant literature). For this, two searches have been carried out in each of the three databases selected by criteria of scientific relevance: Web of Science; Core Collection, and Scopus as the main repositories of indexed scientific production, and Google Scholar. The inclusion of the latter responds to a criterion of openness (greater diversity of scientific production formats) and integration (Martín-Martín et al. (2017) have pointed out the integrating nature of Google Scholar compared to the previous two).
The two searches for each database were performed between September 6th and 12th, 2021 according to the following equations: (“AI”OR“Artificial Intelligence”)AND“Advertising”, to obtain results in English and (“IA”OR“Inteligencia Artificial”)AND“Publicidad” to obtain results in Spanish. The preliminary samples obtained have been the following, based on general results and subsequent thematic screening:
Database |
General |
Thematic Screening |
Advertising |
Marketing |
WoS |
105 |
29 (27,61 %) |
22 (75,86 %) |
7 (24,14 %) |
Scopus |
80 |
39 (48,75 %) |
25 (64,10 %) |
14 (35,9 %) |
Google Scholar English |
324 |
45 (13,88 %) |
32 (71,11 %) |
13 (28,9 %) |
Google Scholar Spanish |
146 |
7 (4,79 %) |
5 (71,4 %) |
2 (28,6 %) |
Source: Own elaboration.
For the general results, any category other than communication, business, economics, and informatics has been excluded. Subsequently, in the thematic screening, those results that were not articulated around the thematic centrality of advertising or commercial communication concerning AI, with thematic approaches such as health, public administrations, legislation, commerce (electronic or conventional), supply chains, value chains, programming, journalism, misinformation, or fiction have been excluded. Those results that, from the perspective of marketing, addressed the impact of AI in advertising communication have been taken into account. It has been verified, according to Martín- Martín et al. (2017), that the sample obtained in Google Scholar subsumes and extends the results obtained in Web of Science and Scopus.
Once the selection was made, a relevant sample of 52 articles was obtained: 45 for the search in English and 7 for the search in Spanish. In the first case, 71.11% of the articles dealt with the subject from the perspective of advertising or commercial communication, and 28.89% did so from the perspective of digital marketing and brand communication. The case of the sample in Spanish presents a similar correlation, with percentages of 71.4% and 28.6%, respectively. The selection has been complemented with professional reports and commercial publications of the sector, which have made it possible to test the validity and/or viability of some of the proposals included in the research on the subject at the current level. Both the search parameters and the set of articles obtained that make up the sample can be consulted at https://doi.org/10.5281/zenodo.6006952.
The common lines of research on AI and advertising have been contrasted with a battery of in-depth interviews conducted with a sample of 20 expert managers in the digital area of 15 representative companies in the advertising sector in Spain. The interviews were aimed at evaluating the internal perspective on the impact of different innovation vectors on the organization and strategy of advertising actors, and the results have been published in the form of a professional report (Martínez and Aguado, 2019).
In the sections that follow, we present how the emerging research works on the impact of AI in advertising address the definitions of the phenomenon, the innovation vectors of AI in advertising, and the transformations that they trigger in the processes of the sector.
Results
Definitions
The interest in providing definitions that allow delimiting the field of study is typical of an emerging field. The definitions provided, even before the object itself (advertising transformed by the impact of AI), concern the concept of artificial intelligence. Rodgers (2021), for example, compiles in a systematic table up to 27 definitions of the term from disciplines as diverse as computing, psychology, mathematics, engineering, advertising, philosophy, medicine, health, commerce, law, education, and engineering. All of them share the features of automatism, effective problem solving, rational guidance through the application, or development of algorithms with the ability to adapt to the evolution of their task performance, and the intensive use of data (Qin and Jiang, 2019; Kumar et al. 2019; Li, 2019; Albinali and Hamdan, 2020).
In the analyzed context, AI implies, therefore, algorithm systems (or applied software) that allow the development of specific tasks and that have a certain learning capacity from training with data in the practice of tasks, so that they can correct and adapt their performance and improve their results (Mittelstadt et al., 2016). Learning can be supervised or autonomous and is developed through training with data sets (machine learning) or through recognition capacity and decisional autonomy (neural networks and deep learning) (Watts and Adriano, 2021). Traditionally in AI, three types of algorithms are considered according to their functionality (Hill, 2016): Predictive analysis (those that allow obtaining information about what will happen in a process and/or why it will happen that way), prescriptive analysis (those that allow obtaining information about what to do and why in a field of causally related possibilities); and automated decision or action (those that perform autonomous actions that require decision-making without human intervention).
On the other hand, the transformative impact of AI as a vector of innovation is the subject of intense theoretical and analytical production (Allan, 2016; Lee et al., 2019; Paschen et al.), also in the field of communication and the media (Song, 2019). This nature as a factor of innovation is associated, according to Paschen et al. 2020, with the ability to alter the ways and times of production processes and with the ability to generate value from the application of analytical or decision-making techniques and tools.
Hancock et al. (2020, p.1) identify communication mediated by artificial intelligence as that form of communication in which “an intelligent agent operates on behalf of a communicator by modifying, improving, or generating messages to meet certain communication objectives”. In this way, advertising mediated by artificial intelligence is seen as one in which technology intervenes to improve the achievement of communication objectives.
In this sense, three complementary concepts are observed in the analyzed literature regarding the impact of artificial intelligence on the advertising process: synthetic advertising, computational advertising, and smart advertising.
Synthetic advertising assumes an approach more focused on the product of advertising (the advertising message), placing the defining emphasis on “ads created or modified by the alteration with artificial intelligence” (Campbell et al., 2021, p. 1). It encompasses, therefore, the use ofAI toimproveimageediting, creation, and post-production processes (as in the case of so-called deepfakes) as well as the incorporation of technologically advanced image formats, such as virtual reality (VR) or augmented reality (AR).
Computational advertising, on the other hand, delimits an approach focused on the medium or tool. The concept designates a broad spectrum of phenomena around the confluence of systems, technologies, and computational methods in advertising:
Refers to an advertising ecosystem with fruitful computing capabilities that employ mathematical, physical, IT, and economic approaches to expressively represent a rich set of advertising objects and environments, model and analyze complex stakeholder behaviors, facilitate efficient, profitable delivery of advertising information to potential consumers across various media vehicles in different contexts of customer/product/brand/firm interactions and touchpoints, and provide efficient, optimal solutions for advertising decision making and market design. (Yang et al. 2017, p. 3).
The concept of computational advertising connects the three basic dimensions of advertising innovation: formats, data, and algorithms, and encompasses all the actors and interactions of its defining processes (Duan and Yang, 2018; Helberger et al., 2020).
The third approach takes a similar line, although more focused on technology and its ability to be customized. Qin and Jiang (2019) identify smart advertising with the application of AI to the design, production, management, and analysis of advertising content. Synthetically, Li (2019:334) defines intelligent advertising as a brand or product/service communication focused on the consumer, based on data exploitation, and mediated by intelligent algorithms. For some researchers, intelligent advertising constitutes a true subdiscipline of advertising that is located “at the intersection between cognitive sciences, computer sciences, and advertising” (Rodgers, 2021: 5). In any case, intelligent advertising constitutes a new phase of digital advertising, after the phase of interactive advertising and that of programmatic advertising (understood as automated buying and selling), marked respectively by the innovation of the Internet and Big Data (Li, 2019). Those texts in the sample that consider the impact of AI in the production and uses of the advertising image with immersive formats such as VR or AR maintain, however, the notion of smart advertising linked to the ability to personalize (Campbell et al., 2021).
It is worth underlining the close connection between data-driven advertising and smart advertising (Yang et al., 2017). The relevance of data processing in nuclear phases of the advertising process, such as the identification of insights, segmentation or research, and control of results, supports a natural connection between the contribution of Big Data (Skiera, 2016; Malthouse and Li, 2017) and the subsequent demand for increasing computing and automation capabilities that pave the way for AI (Chen et al. 2019). But this does not mean that other more qualitative processes of advertising activity, such as creativity, writing, or interaction with the user, are exempt from the disruptive influence of AI.
Innovation vectors
The synthesis or classification of innovation vectors derived from AI in advertising is another point of shared interest in the analyzed literature that explores the limits and scope of new advertising (Helberger et al., 2020). In this sense, innovation vectors are understood as those instrumental features of AI that have a transformative effect on advertising processes (Paschen et al., 2020) and would come to answer the question: what features or capabilities of AI produce innovation in the advertising ecosystem?
Precisely, the efficiency in the processing of large data sets and the identification of relevant patterns constitute one of the instrumental aspects of the contribution of AI that characterize its transformative condition (Kumar and Shaphali, 2016). The analytical power of artificial intelligence allows Big Data to be incorporated into prospective advertising processes, which require identification of objects (insights, profiles...) and decision-making (production, media purchase), particularly regarding unstructured data, which -unlike the structured data with which conventional marketing and advertising work- constitute the true source of value in the digital context (Kietzmann et al., 2018).
The impact on decision-making also includes the areas of creativity or strategic planning, transforming traditionally hierarchical processes, such as the decision on actors or prescription figures (celebrities), production logistics (outdoors), etc. (Lee and Cho, 2019). Both predictive and prescriptive analysis support a radical change in recommendation and segmentation capabilities (Enache, 2020). A change that contributes to transforming the processes of advertising in the interactions between key actors (users, agencies, and the media) but that also facilitates the emergence of new actors in the advertising ecosystem (consultancies, technological intermediaries) (Lee and Cho, 2019).
The ability to automate tasks, and optimize times and processes, is clearly identified in the literature as the second disruptive contribution of AI to advertising (Li, 2019). This contribution is particularly visible in the media planning and sales processes, which, with the development of programmatic purchases, allow managing the coupling of large inventories of spaces and networks of advertisers in real-time, making it possible to adapt the message to the user profile and situation (Busch, 2019). AI- induced acceleration sometimes makes it possible to merge processes that were previously consecutive and belonged to different phases (for example, between the creative and media buying phases, or between the printing and impact evaluation phases). The consequence of the acceleration induced by the automation of tasks is, therefore, that of a reorganization of the processes based on certain simultaneity loops that allow the feedback of decisions to be incorporated in real-time (Qin and Jiang, 2019; Enache, 2020; Ahn, 2020; Rodgers, 2021).
The analyzed texts also point to two areas of development characteristic of AI as sources of growing influence in the field of brand and product communication: complex object recognition capabilities, especially in the case of Natural Language Processing (NLP) and Image Recognition (IR) systems.
Intelligent systems for natural language processing (NLP) are essential for the development of automation of writing tasks (Qin and Jiang, 2019). They also constitute the basis for the development of language recognition apps that make it possible, for example, to effectively explore conversations on social networks to identify insights (Kietzmann et al., 2018) or transform interaction processes with users through conversational algorithms and chatbots, of particular interest in brand communication actions (Li, 2019). Natural language processing capabilities have also proven to be a useful resource in the automated management of the semantic interrelationship between ads and their context, an issue that has traditionally concerned advertising due to the consequences that an inappropriate context could have on the interpretive effectiveness of ads (Watts and Adriano, 2020).
Image recognition (IR), for its part, currently has relevant implications in the creative tasks of advertising: ontheonehandintheoptimizationofprocesses(imageinventorymanagement,editingandpost-production tools, etc.) and on the other, on the optimization of the effectiveness of creativity, making possible forms of automation and personalization of creative processes that allow adapting the image of the ad to the strategy of the advertiser/brand or to the user’s profile (from complementary aspects, such as color, to substantial issues such as the brand or the product itself) (Lee and Cho, 2019; Vrakatsas and Wang, 2020).
The transformation of the advertising process
The third focus of attention of the analyzed articles emphasizes how AI transforms the processes and phases of advertising and would include those contributions of the analyzed sample that answer the question: what aspects of advertising does the impact of AI transform and how does it transform them?
Qin and Jiang (2019) state that smart advertising is instrumental in restructuring advertising processes, including advertising research, strategic planning, creativity, copywriting, media buying, and control. Similarly, Li (2019) identifies insight discovery, ad design and creation, media buying, and campaign effectiveness evaluation as areas of advertising substantially transformed by artificial intelligence.
AI allows advertising research (discovery of insights and consumer trends) and market analysis to be merged in a single step (Qin and Jiang, 2019). The use of language recognition tools to explore activity on social networks in real-time has considerably improved the handling of structured data characteristic of conventional marketing and has led to an unprecedented qualitative leap in the treatment of unstructured data (Skiera, 2016).
Speech and Image Recognition Systems have made it possible to integrate the flow of unstructured data that circulates in the photos, videos, and audio that we share on social networks to the wealth of structured data built from the textual exchanges in which users participate. And, at the same time, the application of AI to the processing of this data makes it possible to identify relevant patterns and generate coherent profiles, which constitute the raw material for the use of predictive analytics on consumer behavior (Shumanov, Cooper, and Ewin, 2021). Kietzman et al. (2018) illustrate, for example, how the digital marketing company Astro uses Microsoft’s Azure system to process billions of data points in a profiling system that allows it to dynamically adapt its clients’ websites in real-time.
Although it is an environment little associated with the impact of AI, its innovative influence is also becoming more noticeable in advertising production and creativity. Several authors (Qin and Jiang, 2019; Vakratsas and Wang, 2020) point to smart copywriting systems as a specific case, which allows the writing phases to be automated and generate an adaptable inventory of slogans, claims, or significant elements of the advertising message.
Through the use of prescriptive algorithms, AI proposes a horizon of optimization of the adaptation of formats and advertising channels to the product, brand, or strategy (Lee and Cho, 2019). This makes it possible to improve decision-making on what type of advertising (outdoor digital, conventional display, desktop, smartTV, mobile, advergame, social networks, voice assistants...) is more appropriate for a certain brand, a specific product or service, or a specific campaign.
AI also makes it possible to enhance the adaptation of the advertising message to the recipient’s profile in real-time, not only in terms of message/user matching (what programmatic buying deals with) but also in terms of dynamic adaptation of the type and content of the message through Dynamic Creative Optimization (DCO) systems (Koren et al. 2020).
DCO systems establish a series of basic combinations between digital advertising message options (colors, aspect, image, text) structured in templates and profiles available through Data Management Platforms (DMP), which take into account basic variables such as time, weather, location, device, ad placement, demographics, and retargeting data.
In this way, a certain flexibility is achieved in adapting the message to the user and its context. This makes it possible, for example, to optimize product recommendation and inventory management systems by adapting the image of each product in the catalog to the user’s profile, as is the case with the covers of the Netflix series and movie catalog, which change their appearance depending on the user’s previous consumption (Song, 2019).
Perhaps the best-known aspect of the impact of AI in advertising is that of programmatic buying, which is leading to a profound transformation of the traditional media buying and planning system (Busch, 2019).
Programmatic buying designates the automation of the process of allocating advertising space in the media as a result of the activity of Ad Exchange networks in which two intelligent systems are involved: Data Management Platforms (DMP) and Demand Side Platforms (DSP). The agencies use the gigantic data collection of the DMPs to evaluate the strategic and opportunity potential of each advertising impression and bid for them through the intelligent algorithms that make up the DSPs (Carrillo-Durán and Rodríguez-Silgado, 2018).
The dominant form of programmatic buying is Real-Time Bidding (RTB), in which advertising networks bid in open auctions for advertising space in real-time, in such a way that the resulting impression in the media is adapted to the specific user profile (Martinez et al., 2017).
Programmatic Direct Buying (PDB) is the other automation format used in AI-powered ad exchange networks. In this case, the purchase is about a select inventory of impressions and media in Private Marketplaces (PMP) that imply a higher quality of data and impressions (Qin and Jiang, 2019). Figure 1 illustrates the ad path in a mobile environment in the context of a real-time auction (RTB).
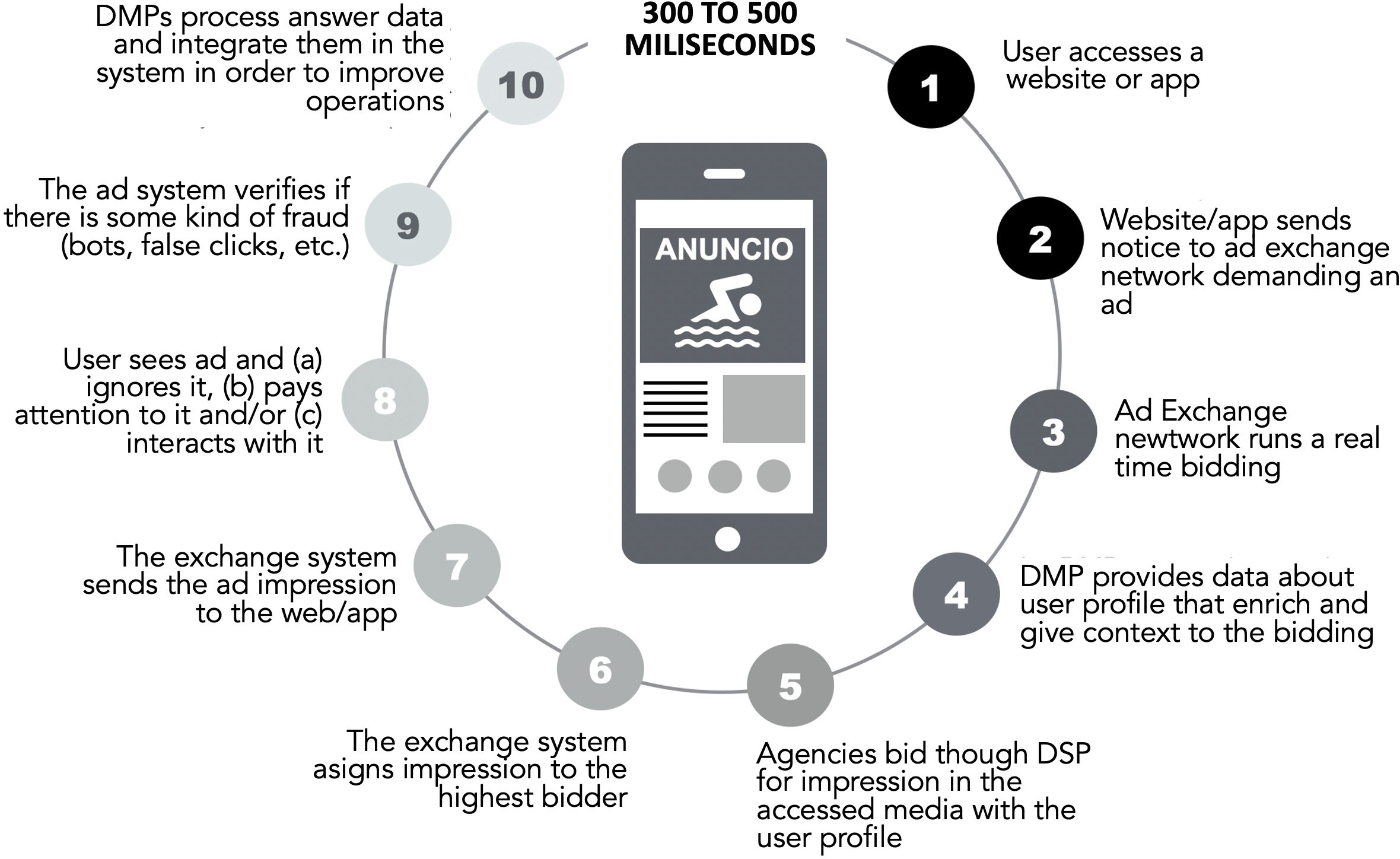
Source: Own elaboration from Busch, 2019.
At this point it is worth highlighting the role of AI in the development of programmatic buying on television and video, where the complexity of handling unstructured data derived from the use of images and audio and its reconciliation with the logic of real-time processes poses some technical challenges of enormous scope (Malthouse et al., 2018).
Based on the potential for automation and exploitation of data repositories that AI offers, Chen et al. (2019) identify an extension of the programmatic buying model to production and creativity processes that extends the capabilities of Dynamic Creative Optimization (DCO) systems. The so-called programmatic creativity, thus, comes to form a new field of optimization coordinated with Content Management Platforms (CMP) that integrates the DCOs with Programmatic Advertisement Creation (PCA) systems. The result is a new programmatic advertising ecosystem that unifies the automation of creativity and media buying and selling (Chen et al. (2019) according to the scheme in Figure 2.
The optimization of processes derived from AI maximizes, in this case, scalability and adaptation in real-time: The ability to manage large repositories of combinable elements for the creation of advertising content allows managing large advertising inventories, as occurs in the case of the operator Verizon, which uses DCO and content management platforms to optimize its native advertising service (Koren et al., 2020). At the same time, the ability to adapt in real-time the appearance and content of the advertising message to the profile and context of the audience allows for improving response rates and the effectiveness of advertising communication (Vrasatkas and Wang, 2020), interconnecting in real-time creativity with impact analysis in a loop that is one of the touchstones of advertising effectiveness (Qin and Jiang, 2019).
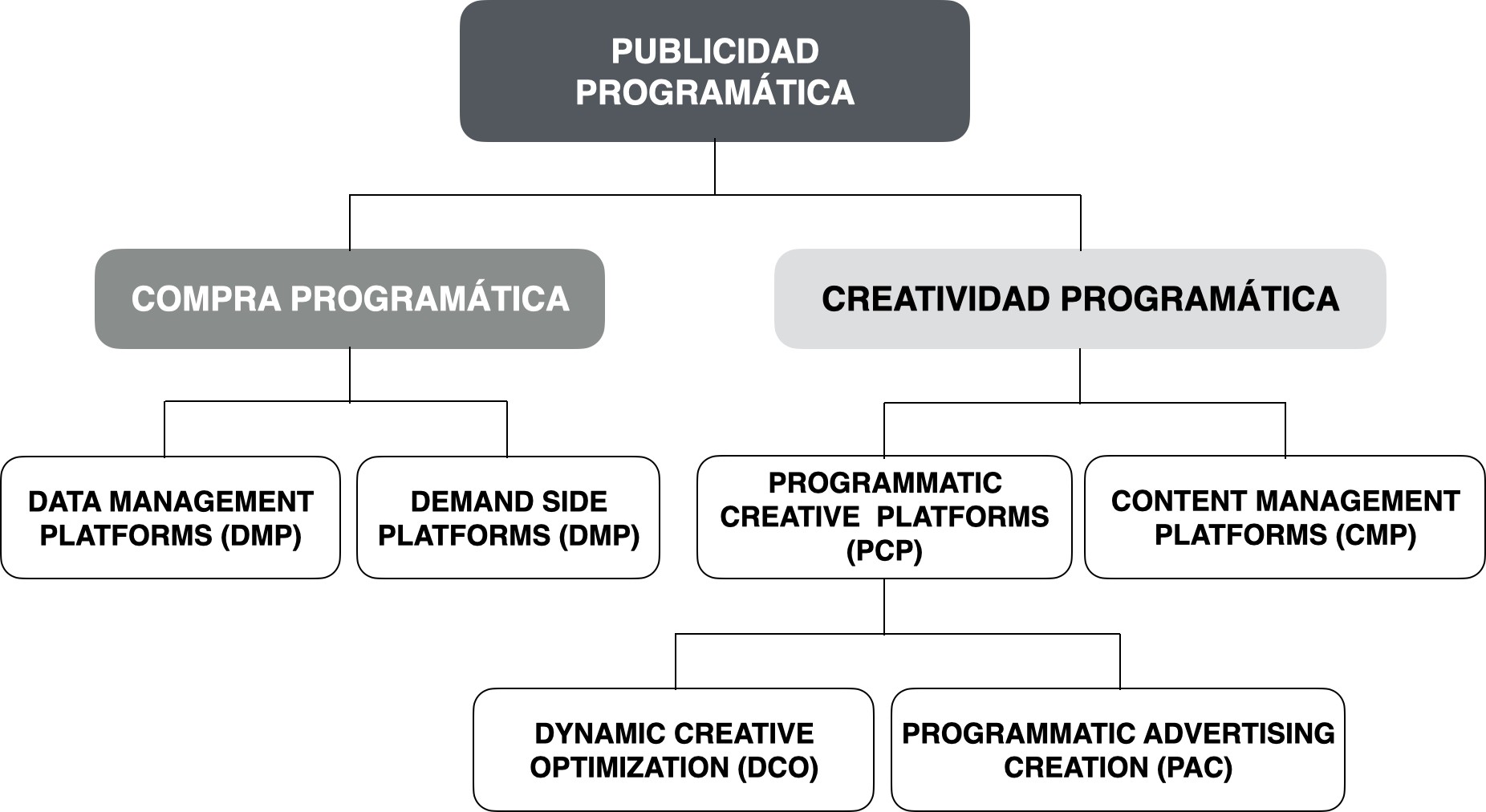
Source: Chen et al., 2019.
In the sphere of the creation of complex images, the fusion between creation, impression and impact evaluation also has implications in the field of product placement. First, the increase in real-time image creation and video editing capabilities using AI expands the horizon of adaptation possibilities. The Microsoft-linked firm BEN (Branded Entertainment Network) uses AI to identify the best scenes and contexts of an audiovisual piece for product placements based on the brand or campaign. And the technology company Mirriad has developed in-content advertising platforms capable of identifying surfaces of objects in the image that may contain advertising or brands (Bradley, 2021). The conjunction between image processing technology, on the one hand, and the identification of profiles in real-time, on the other, allows, for example, to artificially “insert” an ad in a street marquee that a character from a movie crosses or change the beer that the protagonist of a series drinks in an episode to place the product or message that best suits the viewer’s profile (Campbell et al., 2021).
AI also makes it possible to apply facial image reconstruction technology (deepfake) to the creation of ads, resurrecting deceased actors or celebrities to turn them into current advertising prescribers, as happened in 2020 in Spain with the Cruzcampo campaign that used a deepfake of the singer Lola Flores (Palomo-Domínguez, 2021). But the key to its potential, beyond the spectacular nature or the effectiveness of the images created with AI, is that these systems can learn to improve their performance by integrating the analysis of their activity and the feedback derived from the user’s response. (Li, 2019).
The campaign evaluation process in terms of impact and effectiveness is also affected by the disruptive impact of AI. The automation of the processes and their execution in real-time allows you to obtain feedback on the campaigns at the very moment of their implementation, offering the possibility of making strategic decisions and optimizing the campaign on the go. Intelligent performance data processing and analysis systems “reduce the time between impact assessment and campaign optimization to a matter of seconds” (Qin and Jiang, 2019, p. 6). This is, perhaps, the most relevant disruptive element that AI brings to the advertising process: reinserting or merging the evaluation of effectiveness or impact as one more element of each phase. For the sample addressed, the fusion between execution and measurement constitutes the true revolution of AI in its contribution to the organizational dynamics of advertising (Lee et al., 2019). In this sense, the internal perception of the actors coincides with the processes that the research works reflect, pointing in both cases to AI and Big Data as the two engines that determine the recent evolution of the sector (Martínez and Aguado, 2019).
Beyond the traditional advertising process, the consulted literature also includes a potential niche for the application of AI tools and processes in the field of brand communication: the use of chatbots or conversational algorithms in social networks opens up the possibility, according to Thomas and Fowler (2020), for the development of artificial prescribers or AI influencers. Artificial conversations are already an important part of social networks: In a study on the presence of bots on Twitter, Liu (2019) has identified that at least 30% of the content on the social network is produced by algorithms. At the same time, companies are beginning to experiment with virtual influencers who embody brand values associated with the identity of their followers. According to Rodrigo-Martín et al. (2021), virtual influencers are “avatars created using artificial intelligence and augmented reality techniques that allow the communication and advertising possibilities of the traditional influencer to be combined with absolute control of their activity and “life” on social networks”. Rodrigo-Martin et al. (2021) point to the case of Lil Miquela, a 19-year-old fashion influencer, musician, and model who defines herself as queer and a Black Lives Matter activist, who has more than three million followers on Instagram, and who, in reality, is a virtual avatar created and managed by Brud, a Los Angeles technology company. The potential of AI in this field, as Thomas and Fowler (2020) point out, goes beyond the creation and characterization of the avatar, to include the adaptation of the behavior and the message of the algorithmic prescribers to the profiles and personality of their followers, thus maximizing the effectiveness of the prescription.
Discussion
The analysis carried out allows us to point out a classification in categories of the lines of research outlined in the current literature on the impact of AI in advertising. Table 2 shows how the descriptive and instrumental orientation of this first wave of publications on the subject privileges the conventional phases of the advertising process and its transformation in terms of procedure and effectiveness.
Some complementary lines of research of the traditional advertising process appear along with them, although collaterally. Here the attention paid to innovations in image processing and recognition and their implications in the so-called synthetic advertising (Campbell et al., 2021), the research of its consequences in the product placement strategy (ibid.), and the creation of artificial actors (Palomo- Domínguez, 2021) stand out. In the specific territory of brand communication, there is also a growing interest in the application of the conversational capabilities of AI, expressed in the development of artificial prescribers such as virtual influencers (Rodrigo-Martín et al., 2021).
Advertising phases |
MARKET RESEARCH AND ANALYSIS |
STRATEGIC PLANNING AND CREATIVITY |
MEDIA PLANNING AND BUYING |
EVALUATION OF IMPACT AND EFFECTIVENESS |
Applied to |
Social network analysis |
Strategic decisions |
Programmatic buying |
Impact analysis |
Programmatic Creativity |
Efficacy Metrics |
|||
Image creation |
||||
Instrumental contribution of AI |
Big Data NLP |
Big Data + machine learning |
Big Data |
Big Data + machine learning |
NLP / IR |
Deep learning |
Deep learning |
||
Deep learning |
Neural networks |
|||
Innovation or improvement |
Insights discovery Analysis of consumption trends |
Creativity automation |
Automation of the purchase and planning |
Automation of the evaluation process |
Autonomous strategic decisions in real-time |
Autonomous strategic decisions in real-time |
|||
Augmented image/ synthetic advertising |
Source: Own elaboration from Qin and Jiang, 2019.
The impact of AI in the transformation of the characteristic processes of advertising dynamics is, wi- thout a doubt, the main object of interest in the addressed literature. In fact, the absence in the analyzed research of the organizational transformations of the actors of advertising is striking, precisely when the difficulties of adaptation of the conventional actors are more evident (crisis of the large agencies model, specialization in activity niches, etc.) and the confluence of new actors from the field of tech- nology and data management (Martínez and Aguado, 2019).
The interviews with representative actors of the advertising ecosystem on the impact of digital technology (Ibid.) highlight a different perspective: along with the importance of innovation in processes, the interviewees unanimously point to the transformation of the advertising ecosystem as a derived consequence.
The case of the so-called cookie apocalypse is perhaps the most visible example of this dual implication of AI-induced innovation in advertising. In the summer of 2019, the ICO (Information Commissioner’s Office, the United Kingdom’s Data Protection Control Authority) published a report on digital advertising technology and RTB in which it stated that, although automation of the purchase- sale of online advertising was an irreversible process, the technology and procedures currently used for the implementation of the RTB cast serious doubts on the level of compliance and accountability in terms of privacy (ICO, 2019). According to the ICO, the ad-tech industry had a deliberately diminished perception of privacy risks, obligations, and responsibilities in programmatic advertising processes. At the same time, the EU specified in 2020 the proposal for a Digital Services Law that is articulated as a common set of rules on the obligations of intermediaries and accountability in digital services, with a specific chapter on privacy protection (EU, 2021).
In line with these legislative initiatives, different technological actors (Apple, Firefox, Opera, etc.) announced in 2019 a series of strategic decisions that limit or directly eliminate the use of third-party cookies. Third-party cookies are the main source of data on Internet use. In 2020, Google anticipates that Chrome (with a 66% market share among browsers) will stop using third-party cookies by 2022 (Adform, 2019). The initiative is part of the so-called Chromium Privacy Project, which will use the sandbox model (or isolated processing environments), applying AI to guarantee privacy in transactions between servers (Buckley, 2021).
The abandonment of third-party cookies in the digital advertising ecosystem represents a profound organizational transformation of the online advertising market as we know it today (Barker, 2020). Third-party cookies are the main way of generating data for the creation of profiles that, in turn, allow the automation and micro-segmentation processes on which many of the AI applications in advertising are based. The actors of the new ecosystem will have to adapt to the new conditions and develop technological solutions that allow privacy-friendly identification processes, but the level of uncertainty about a market that is still in formation is high. Also, not all consequences are advantageous for users. For some researchers (Houle and Mauss, 2021), the disappearance of third-party cookies may lead to a “feudalization of the Internet”, that is, to the elimination of small actors that depend entirely on tertiary data (that provided by third-party cookies) to develop their business, instead strengthening the big actors –both media, agencies, and intermediaries- with the capacity to generate large volumes of primary data from their broad user base. As Buckley (2020) has pointed out, the role of AI is key in the development of solutions based on alternative technologies to cookies, less invasive of privacy: the so-called PET (Privacy Enhancing Technologies).
The case of the regulation and development of policies on cookies illustrates the close interrelation between innovation in processes and the transformation of the advertising ecosystem and opens a horizon of lines of research on the impact of AI in advertising that takes into account changes in the internal organization of the actors in the advertising ecosystem, in their interactions, and in the processes through which they generate value and innovation.
Conclusions
Existing research on the impact of AI in advertising is still in its infancy. This translates into a non- specialized general character, which tends to collect contributions from other related fields in which the study of the impact of AI is more developed (fundamentally marketing and online commerce), or from the computer sciences themselves that treat AI from technical standards. The analyzed research works predominantly present a descriptive and modeling character, paying attention to the definitions, the detailed description of the innovative processes, and their exemplification with significant cases.
The descriptive nature of an incipient approach and the preceding literature on the adoption of technological innovations (Allam, 2020) are the most likely cause that, in practically the entire sample, the standard for approaching the analysis is that of the phases of the advertising process and its transformation. In this sense, the analyzed research works go through one, several, or all the moments of the “advertising journey”, from the research of consumer trends and market analysis to the evaluation of the impact of campaigns, passing through strategic planning, message production and creativity, and media buying and planning (Kietzmann et al., 2018; Qin and Jiang, 2019; Lee and Cho, 2019; Li, 2019; Chen et al. 2019; Vkratsas and Wang, 2020). In all cases, the vision of user-centered advertising is proposed, based on the exhaustive and intensive exploitation of data and oriented towards optimization and automation (Kumar and Saphali, 2016), in which the processes are subjected to acceleration and, ultimately, to simultaneity.
The result of this descriptive approach focused on the phases of the advertising process, as noted by Qin and Jiang (2019), is that the advertising process maintains its structure and defining phases, although these are notably optimized in terms of effectiveness and time. Innovation seems to focus, thus, on “new– more effective – ways of doing the usual”, rather than on a profound change in the logic of the ecosystem – the set of actors and their defining interactions. We believe, however, that it is necessary to go beyond the descriptive-instrumental phase that characterizes the research analyzed in two ways:
First, to address the analysis of the impact of AI in the advertising ecosystem, taking into account not only the processes (and how they create value beyond effectiveness) but also the actors involved in those processes and the interrelationships that make them possible (Martínez and Aguado, 2019). It is surprising, in this sense, that none of the research that analyzes the impact of AI in advertising has proposed a review of the internal and external organizational changes of conventional actors, or the appearance of new actors related to technological specialization, regarding the transformation of advertising processes. An analysis of the changes in the value networks of the advertising ecosystem as well as the economic impact of the innovations induced by the incorporation of AI is also pending.
In fact, from the perspective of the consequences of instrumental innovations, the research analyzed tends to show certain myopia typical of the descriptions dependent on the casuistry of the sector, thus favoring short-term productivity in the analysis (hence the emphasis on acceleration, automation, or efficiency). Obviously, with a longer view, things can look very different. In the field of Big Data (the other great vector of innovation) Pärssinen et al. (2018), point out, for example, that, far from solving the problems and challenges of digital advertising, the automation of processes and the exhaustive use of data have contributed to aggravating some of the most dysfunctional dynamics of the advertising ecosystem that have arisen with the digital change:
The online advertising ecosystem - the authors point out - has become infested with thousands of intermediaries, whose business models range from exploiting user data to verification companies promising to help advertisers secure their advertising budgets. The principal parties in online advertising – users, advertisers and publishers – have recently all pointed out concerns related to these intermediaries, the actual value they provide, and how in many cases they operate against the interests of at least one of the above-mentioned key players. (Pärssinen et al., 2018, p. 54885).
Second, it is necessary to include in the research a critical perspective that connects the changes in advertising derived from AI with the framework of the transformations of the media environment and, in particular, with some of the key issues of digital societies, such as privacy (Christiansen, 2011). Along the same lines, subsequent research should address how other characteristic problems of AI impact the advertising ecosystem, such as algorithmic biases or algorithmic opacity, and contribute to the elucidation of algorithmic transparency or accountability formulas in the field of advertising that allows sustainable innovation (Cillo et al., 2019) beyond the conventional criteria of effectiveness and optimization.
The development horizon of the research of the innovativeimpact ofAI in advertising can thus be stratified into three complementary levels: The instrumental level, focused on processes, of a descriptive-analytical nature according to criteria of effectiveness (innovation in objects and their production process); the ecosystemlevel,focusedontheinteractionsbetweenactors,theirinternalorganizationandthedevelopment ofvaluecreationnetworks,ofamodelingnatureaccordingtocriteriaofadaptabilityandviability(innovation in organizational processes and the network of interdependencies); and the level of sustainability of the innovation, which incorporates ethical criteria and connects the disruption with the social context.